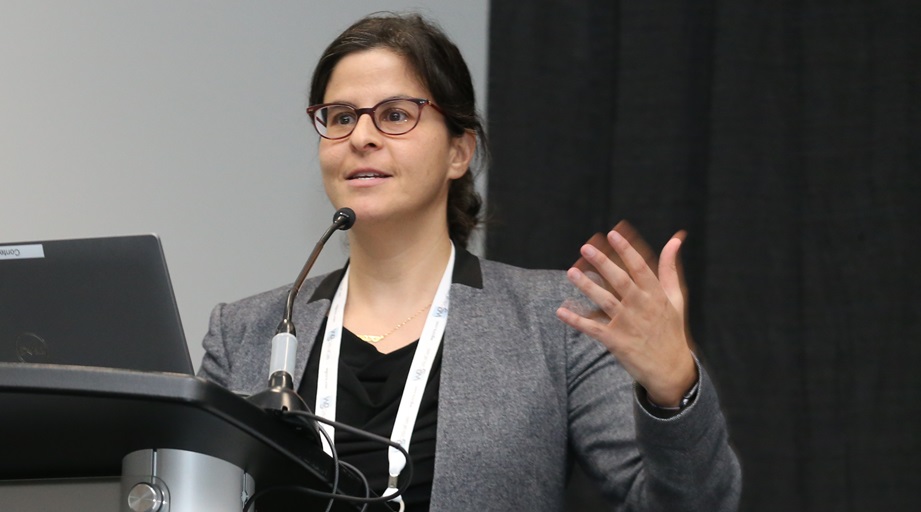
With artificial intelligence (AI) making inroads in drug development, federal regulators must set ground rules that can evolve as quickly as the technology itself, according to Tala Fakhouri, associate director for policy analysis at the Food and Drug Administration (FDA).
“It is our role to be responsive to how the emerging technology is being used. And this is why having static regulations generally doesn’t work,” Fakhouri said at a Dec. 3 session on AI in drug development at the 2023 Midyear Clinical Meeting & Exhibition in Anaheim, California. “That’s why this continuous dialogue with industry is very important when it comes to emerging technologies.”
An Oct. 30 executive order by President Joe Biden instructed federal agencies to develop within a year regulations and standards for AI across different industries. In healthcare, the past decade has been marked by a rapid expansion of AI and machine learning (ML), a subset of AI that gives computers the ability to learn without being programmed.
That can be seen already in practices, where physicians, for example, use technology that records conversations and takes doctors’ notes, said Fakhouri. And it can be seen in medical research. The number of medical studies incorporating AI grew from 4,725 published articles in 2017 to 12,500 in 2019, according to data presented in the session.
AI/ML is still in its early stages in drug development, but drugmakers are making big bets on it. Johnson & Johnson, for example, has hired 6,000 data scientists and digital specialists and pumped hundreds of millions of dollars into their work, including using machines to analyze huge health-record datasets, The Wall Street Journal reported last week.
The FDA’s Center for Devices and Radiological Health, which Fakhouri said is leading the way in crafting AI regulations, has approved 500 medical devices using AI/ML, nearly all of them in the last four years.
“It’s happening, it’s a reality,” she said. “What’s important right now is for all of us in the healthcare setting to make sure it’s being used in a responsible way.”
AI has potential uses across the drug development landscape, from discovery (drug target identification and compound screening) to clinical research (recruitment and data collection) to manufacturing and postmarket safety monitoring.
Seven months ago, the FDA published a discussion paper on AI/ML, noting that it sees substantial benefits for drug development, including helping to bring safe and effective drugs to market much more quickly; improving the quality of manufacturing; and developing novel drugs and personalized treatment approaches.
In clinical research, AI/ML is already being used to analyze data from both clinical trials and observational studies to make inferences about the safety and effectiveness of a drug, according to the FDA. Innovations are also underway to connect patients to trials for investigational treatments by mining data, including clinical trial databases, social media, and medical literature.
Submissions to the FDA from 2016 to 2021 included AI/ML applications to perform a variety of tasks, including enhancing clinical trial design, optimizing dosages, and enhancing adherence to drug regimens, according to an analysis published in April in Clinical Pharmacology & Therapeutics.
But there are risks and shortcomings associated with such innovations, including a scarcity of high-quality datasets, opacity of the algorithms, and data privacy and security, said Fakhouri.
Black box algorithms, for example, pose a particularly difficult problem in healthcare. “If an algorithm is telling a clinician to do A versus B, the clinician wants to know why it told them to do A,” she said. “There has to be some medical knowledge involved in knowing why it came out to that specific decision.”
More than 65 institutions submitted comments to the FDA following the release of the discussion paper. Institutions wanted to know the details of FDA’s proposed oversight. Some of them also called for the establishment of public–private partnerships to advance the creation and sharing of machine-readable data sets for drug development.
During the Q&A session, an audience member pointed out that a current lack of robust data on specific drugs can cause algorithms to produce nonsensical results. Fakhouri said that’s why the human element remains so critical.
“This is one of the challenges, the data-driven nature of these algorithms where you could get someone’s horoscope being associated with a drug working or not working,” she said. “This is why we keep emphasizing human-AI teams, multidisciplinary teams. The hope is that the clinician interference provides some data that could then be used to retrain the models."